Box Plot Diagram in Excel
A box plot diagram, also known as a box-and-whisker plot, is a graphical representation of the distribution of data. It can show the median, quartiles, and outliers of a dataset. Box plot diagrams are often used to compare the distributions of two or more datasets.
To create a box plot diagram in Excel, follow these steps:
- Select the data you want to plot.
- Click on the “Insert” tab.
- Click on the “Chart” button.
- Select the “Box Plot” chart type.
- Click on the “OK” button.
The box plot diagram will be created in the worksheet. The median of the data will be represented by a line in the box. The quartiles will be represented by the edges of the box. The outliers will be represented by points outside of the box.
Box plot diagrams can be a helpful tool for visualizing the distribution of data. They can be used to identify outliers, compare the distributions of two or more datasets, and make inferences about the underlying population.
Benefits of Box Plot Diagrams
- They are easy to create and interpret.
- They can show the median, quartiles, and outliers of a dataset.
- They can be used to compare the distributions of two or more datasets.
- They can help to identify outliers.
Tips for Creating Effective Box Plot Diagrams
- Use clear and concise labels for the axes.
- Make sure the data is sorted in ascending order.
- Use a different color for each dataset.
- Add a legend to explain the meaning of the different symbols.
- Consider using a logarithmic scale if the data is skewed.
By following these tips, you can create effective box plot diagrams that will help you to visualize and understand your data.
Key Aspects of Box Plot Diagrams in Excel
Box plot diagrams are a valuable tool for visualizing and understanding data distributions in Excel. They are particularly useful for comparing multiple datasets and identifying outliers.
- Data Representation: Box plots use a simple and intuitive graphical representation to show the median, quartiles, and outliers of a dataset.
- Distribution Comparison: By comparing the box plots of multiple datasets, we can quickly identify differences in their distributions, such as central tendency, spread, and skewness.
- Outlier Detection: Box plots clearly highlight outliers, which are data points that are significantly different from the rest of the dataset.
- Data Exploration: Box plots can be used as a starting point for further data exploration, such as identifying patterns, trends, and potential relationships between variables.
- Customization: Excel allows for customization of box plot diagrams, including the ability to change colors, add labels, and adjust the scale.
- Interpretation: Understanding how to interpret box plot diagrams is crucial for accurately drawing conclusions from data analysis.
These key aspects highlight the versatility and usefulness of box plot diagrams in Excel. By leveraging these features, we can gain valuable insights into our data, make informed decisions, and communicate our findings effectively.
Data Representation
The data representation aspect of box plot diagrams is crucial for understanding their significance in Excel. Box plots use a simple and intuitive graphical representation to convey key statistical information about a dataset, making them a powerful tool for data visualization and analysis.
The graphical representation of a box plot diagram consists of a box and two whiskers. The box represents the interquartile range (IQR), which is the range between the first quartile (Q1) and the third quartile (Q3). The median, which is the middle value of the dataset, is represented by a line within the box. The whiskers extend from the edges of the box to the most extreme data points within a certain range, typically 1.5 times the IQR. Any data points beyond this range are considered outliers and are plotted as individual points outside the whiskers.
This graphical representation allows for quick and easy visual assessment of the data’s central tendency, spread, and potential outliers. By comparing multiple box plots, we can identify differences in the distributions of different datasets, such as variations in median values, the presence of outliers, and the overall shape of the distributions.
In the context of Excel, box plot diagrams are particularly valuable for analyzing large datasets or comparing multiple datasets simultaneously. They provide a concise and informative visual summary of the data, facilitating data exploration, hypothesis testing, and decision-making.
In summary, the data representation aspect of box plot diagrams is fundamental to their usefulness in Excel. The simple and intuitive graphical representation allows us to quickly visualize and understand the median, quartiles, and outliers of a dataset, enabling effective data analysis and informed decision-making.
Distribution Comparison
The ability to compare the distributions of multiple datasets is a critical aspect of box plot diagrams in Excel. By visually comparing the box plots, we can quickly identify differences in the central tendency, spread, and skewness of the underlying data.
Central tendency refers to the middle or average value of a dataset. Box plots allow us to compare the median values of multiple datasets, which provides a more robust measure of central tendency than the mean, especially when dealing with skewed data. By observing the positions of the median lines within the boxes, we can determine which datasets have higher or lower central values.
Spread refers to the variability or dispersion of data points within a dataset. Box plots help us visualize the interquartile range (IQR), which represents the middle 50% of the data. By comparing the lengths of the boxes, we can assess the spread of the data and identify datasets with greater or lesser variability.
Skewness refers to the asymmetry of a distribution. Box plots can reveal skewness by showing whether one whisker is significantly longer than the other. A longer whisker indicates a greater number of data points in that direction, providing insights into the shape of the distribution.
The practical significance of understanding distribution comparison using box plot diagrams in Excel lies in its wide range of applications. For example, in financial analysis, comparing the box plots of stock prices over time can help identify trends, volatility, and potential investment opportunities. In healthcare, comparing the box plots of patient outcomes for different treatments can aid in evaluating treatment effectiveness. In manufacturing, comparing the box plots of product quality metrics can assist in identifying areas for improvement.
In summary, the distribution comparison aspect of box plot diagrams in Excel is a powerful tool for visually comparing the central tendency, spread, and skewness of multiple datasets. By leveraging this capability, we can gain valuable insights into the underlying data, make informed decisions, and communicate our findings effectively.
Outlier Detection
Outlier detection is a critical aspect of box plot diagrams in Excel, as it allows us to identify data points that deviate significantly from the rest of the dataset. These outliers can provide valuable insights into the underlying data and may represent errors, anomalies, or unique characteristics that require further investigation.
- Identification: Box plots use a simple and effective method to identify outliers. Data points that lie outside the whiskers, which typically extend 1.5 times the interquartile range (IQR) from the edges of the box, are considered outliers.
- Visual Representation: Outliers are plotted as individual points outside the whiskers, making them visually distinct from the rest of the data. This visual representation allows for quick and easy identification of outliers, even in large datasets.
- Data Exploration: Identifying outliers can be a starting point for further data exploration. By examining the outliers, we can gain insights into potential errors in data collection or entry, unusual observations, or unique characteristics of the dataset.
- Decision-Making: Outliers can influence statistical analysis and decision-making. Understanding the presence and potential causes of outliers can help us make informed choices about data handling, model selection, and interpretation of results.
In the context of Excel, box plot diagrams are particularly useful for outlier detection in large datasets or when comparing multiple datasets. By visually identifying outliers, we can gain a better understanding of the overall distribution of the data and make more informed decisions.
In summary, the outlier detection aspect of box plot diagrams in Excel is a valuable tool for identifying data points that deviate significantly from the rest of the dataset. By visually representing outliers, box plots facilitate data exploration, enhance decision-making, and provide insights into the underlying data.
Data Exploration
Box plots are a powerful tool for data exploration, providing a visual representation of the distribution of data and highlighting potential patterns, trends, and relationships. By examining box plots, we can gain insights into the central tendency, spread, skewness, and presence of outliers in the data.
This information can serve as a starting point for further data exploration, leading to a deeper understanding of the underlying relationships and patterns within the data. For example, by comparing box plots of different groups or categories, we can identify differences in distributions and explore potential causes for these differences.
Furthermore, box plots can help identify potential relationships between variables. By examining the positions and shapes of box plots for different variables, we can visually assess whether there are any correlations or associations between them. This can lead to the formulation of hypotheses and further statistical analysis to confirm or refute these relationships.
In the context of Excel, box plot diagrams are particularly useful for data exploration due to their ease of creation and interpretation. The visual representation provided by box plots makes it quick and easy to identify patterns and trends, even in large datasets.
In summary, the data exploration aspect of box plot diagrams in Excel is a valuable tool for gaining insights into the distribution and relationships within data. By visually representing the data, box plots facilitate the identification of patterns, trends, and potential relationships, serving as a starting point for further data exploration and analysis.
Customization
The customization capabilities of box plot diagrams in Excel enhance their utility and effectiveness in data analysis and visualization. Excel provides users with the flexibility to tailor box plots to meet specific requirements and preferences, ensuring clear and informative graphical representations of data.
Changing Colors: Color customization allows users to assign different colors to the boxes, whiskers, and outliers, making it easier to visually distinguish between multiple datasets or categories. This customization is particularly useful when comparing multiple box plots on the same graph, as it improves visual clarity and facilitates quick identification of patterns and trends.
Adding Labels: Adding labels to box plots provides additional context and information to the visualization. Users can add labels to the axes, title, and legend to clearly describe the data being represented. This customization enhances the interpretability of the box plot and makes it easier for viewers to understand the key findings.
Adjusting the Scale: Excel allows users to adjust the scale of the box plot, including the axis limits and tick marks. This customization is crucial when dealing with data that has a wide range or when comparing box plots with different scales. By adjusting the scale appropriately, users can ensure that the data is represented accurately and that comparisons between box plots are valid.
The practical significance of customization in box plot diagrams extends to various fields and applications. For example, in financial analysis, customizing box plots allows analysts to highlight key metrics and compare the performance of different stocks or investments. In healthcare, customized box plots can be used to visualize patient outcomes and identify trends or differences between treatment groups. In manufacturing, customization enables engineers to compare quality metrics and identify areas for improvement.
In summary, the customization capabilities of box plot diagrams in Excel empower users to create tailored visualizations that meet their specific needs. By changing colors, adding labels, and adjusting the scale, users can enhance the clarity, interpretability, and effectiveness of their box plots, leading to more informed decision-making and better communication of data insights.
Interpretation
Interpretation is a fundamental aspect of box plot diagrams in Excel, as it allows us to draw accurate conclusions from the data being visualized. Box plot diagrams provide a concise and informative representation of data distribution, but their effectiveness relies on the ability to correctly interpret the graphical elements.
To interpret a box plot diagram effectively, it is important to understand the following key components:
- Median: The median line within the box represents the middle value of the dataset, dividing the data into two equal halves.
- Interquartile Range (IQR): The box represents the IQR, which is the range between the first quartile (Q1) and the third quartile (Q3).
- Whiskers: The whiskers extend from the edges of the box to the most extreme data points within a certain range, typically 1.5 times the IQR.
- Outliers: Data points that lie outside the whiskers are considered outliers and are plotted as individual points.
By understanding these components, we can interpret the box plot diagram to gain insights into the central tendency, spread, skewness, and presence of outliers in the data. This interpretation is crucial for accurately drawing conclusions from data analysis.
For example, in financial analysis, interpreting box plot diagrams of stock prices can help investors identify trends, volatility, and potential investment opportunities. In healthcare, interpreting box plot diagrams of patient outcomes can aid in evaluating treatment effectiveness and identifying areas for improvement. In manufacturing, interpreting box plot diagrams of product quality metrics can assist in identifying defects and improving production processes.
In summary, the interpretation of box plot diagrams is essential for accurately drawing conclusions from data analysis. By understanding the key components and their significance, we can effectively use box plot diagrams to gain valuable insights into the underlying data, make informed decisions, and communicate our findings clearly.
A box plot diagram, also known as a box-and-whisker plot, is a graphical representation of the distribution of data. It is a standardized way of displaying the five-number summary of a dataset: the minimum, first quartile, median, third quartile, and maximum.
Box plot diagrams are useful for visualizing the spread and central tendency of data, and for comparing the distributions of two or more datasets. They are widely used in a variety of fields, including statistics, finance, and manufacturing.
In Microsoft Excel, box plot diagrams can be easily created using the built-in charting tools. This makes it a convenient and accessible tool for data analysis and visualization.
FAQs on Box Plot Diagrams in Excel
Box plot diagrams are commonly used for visualizing and comparing data distributions in Excel. Here are some frequently asked questions (FAQs) about box plot diagrams in Excel:
Question 1: What does a box plot diagram show?
A box plot diagram shows the five-number summary of a dataset: minimum, first quartile, median, third quartile, and maximum. It also displays the interquartile range (IQR) and outliers.
Question 2: How do I create a box plot diagram in Excel?
You can create a box plot diagram in Excel by selecting the data you want to plot, then clicking on the “Insert” tab and selecting “Box Plot” from the charts section.
Question 3: How do I interpret a box plot diagram?
To interpret a box plot diagram, look at the median line, the IQR, and the presence of outliers. The median line indicates the middle value of the dataset, the IQR shows the spread of the middle 50% of the data, and outliers are data points that are significantly different from the rest of the dataset.
Question 4: How can I customize a box plot diagram in Excel?
You can customize the appearance of a box plot diagram in Excel by changing the colors, adding labels, and adjusting the scale.
Question 5: What are the benefits of using box plot diagrams?
Box plot diagrams are beneficial for visualizing the distribution of data, comparing multiple datasets, identifying outliers, and making data-driven decisions.
Question 6: When should I use a box plot diagram?
Box plot diagrams are appropriate when you want to get a quick overview of the distribution of data, compare multiple datasets, or identify outliers.
In summary, box plot diagrams are a versatile and powerful tool for data visualization and analysis in Excel. By understanding how to create and interpret box plot diagrams, you can gain valuable insights into your data.
Stay tuned for the next section, where we will provide practical tips and best practices for creating effective box plot diagrams in Excel.
Conclusion
In conclusion, box plot diagrams are a powerful tool for visualizing and analyzing data distributions in Microsoft Excel. They provide a clear and concise representation of the five-number summary, interquartile range, and outliers, making them ideal for exploring data, comparing multiple datasets, and identifying patterns and trends.
By leveraging the customization options available in Excel, users can create tailored box plots that meet their specific requirements and preferences. This flexibility enhances the interpretability and effectiveness of box plots, ensuring that they can be used to communicate data insights effectively.
As data analysis becomes increasingly important in various fields, box plot diagrams will continue to be a valuable asset for data scientists, analysts, and professionals across industries. Their simplicity, versatility, and informative nature make them a go-to choice for data visualization and exploration.
Youtube Video:
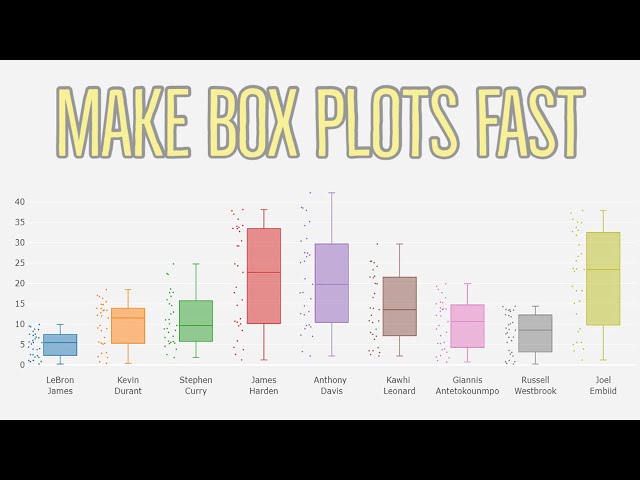