Data science practice projects are hands-on applications of data science concepts and techniques to solve real-world problems. They involve collecting, cleaning, analyzing, and interpreting data to extract insights and make informed decisions.
These projects are essential for data scientists as they provide an opportunity to apply their skills in a practical setting, develop their problem-solving abilities, and enhance their understanding of the data science process. They also allow data scientists to showcase their work and demonstrate their proficiency in the field.
Data science practice projects can cover a wide range of domains, such as healthcare, finance, marketing, and manufacturing. They can involve tasks such as predictive modeling, anomaly detection, natural language processing, and image recognition. By working on these projects, data scientists can gain valuable experience and prepare themselves for the challenges of the real world.
Data Science Practice Projects
Data science practice projects are essential for data scientists as they provide an opportunity to apply their skills in a practical setting, develop their problem-solving abilities, and enhance their understanding of the data science process. They also allow data scientists to showcase their work and demonstrate their proficiency in the field.
- Hands-on experience: Practice projects provide data scientists with hands-on experience in the entire data science process, from data collection to analysis and interpretation.
- Problem-solving skills: Practice projects challenge data scientists to solve real-world problems using their data science skills.
- Domain knowledge: Practice projects allow data scientists to apply their skills to specific domains, such as healthcare, finance, or marketing.
- Communication skills: Practice projects help data scientists develop their communication skills by requiring them to present their findings to stakeholders.
- Collaboration skills: Practice projects often involve collaboration with other data scientists and team members, fostering teamwork and communication skills.
- Portfolio building: Practice projects provide data scientists with valuable experience andthat they can add to their portfolio to showcase their skills.
These aspects are essential for data scientists as they help them develop the skills and experience needed to be successful in the field. By working on practice projects, data scientists can gain valuable insights into the data science process and its applications in various domains.
Hands-on experience
Hands-on experience is a crucial component of data science practice projects, as it allows data scientists to apply their theoretical knowledge to real-world problems and gain a deeper understanding of the data science process. Through practice projects, data scientists can experience the entire data science lifecycle, from data collection and cleaning to analysis and interpretation, which helps them develop a comprehensive understanding of the field.
For example, a data scientist working on a practice project to predict customer churn may need to collect data from various sources, such as customer surveys, transaction records, and website logs. They would then need to clean and prepare the data, identify relevant features, and build a predictive model. By working through this process hands-on, the data scientist gains valuable experience in each step of the data science pipeline.
Overall, hands-on experience is essential for data scientists as it allows them to develop the skills and knowledge needed to be successful in the field. Practice projects provide a valuable opportunity for data scientists to gain this experience and enhance their understanding of the data science process.
Problem-solving skills
Data science practice projects play a critical role in developing problem-solving skills among data scientists. These projects present data scientists with real-world challenges that require them to apply their knowledge and skills to find solutions. By working on these projects, data scientists can develop their ability to identify and define problems, gather and analyze data, and develop and implement solutions.
- Critical thinking: Practice projects require data scientists to think critically about the problem they are trying to solve and the data they have available. They need to be able to identify the key variables, assumptions, and constraints involved in the problem.
- Analytical skills: Practice projects also require data scientists to have strong analytical skills. They need to be able to analyze data from multiple sources, identify patterns and trends, and draw conclusions from the data.
- Problem-solving: Once data scientists have analyzed the data, they need to be able to develop and implement solutions to the problem. This may involve building predictive models, developing algorithms, or creating visualizations.
- Communication skills: Practice projects also help data scientists develop their communication skills. They need to be able to communicate their findings to stakeholders in a clear and concise manner.
Overall, data science practice projects are essential for developing problem-solving skills among data scientists. These projects provide data scientists with the opportunity to apply their knowledge and skills to real-world problems and develop the critical thinking, analytical, and problem-solving skills necessary to be successful in the field.
Domain knowledge
Domain knowledge is essential for data scientists as it allows them to understand the specific challenges and opportunities within a particular industry or field. By working on practice projects in a specific domain, data scientists can develop a deep understanding of the data, the business processes, and the key stakeholders involved. This knowledge enables them to develop more effective and tailored data science solutions.
For example, a data scientist working on a practice project in the healthcare domain may need to understand medical terminology, disease progression, and treatment protocols. This knowledge would allow them to develop more accurate and reliable predictive models for disease diagnosis and prognosis. Similarly, a data scientist working on a practice project in the financial domain may need to understand financial markets, investment strategies, and risk management. This knowledge would allow them to develop more robust and profitable trading algorithms.
Overall, domain knowledge is a critical component of data science practice projects, as it allows data scientists to develop more effective and tailored solutions for specific industries and fields. By gaining domain knowledge, data scientists can increase the impact and value of their work.
Communication skills
Communication skills are essential for data scientists as they need to be able to effectively communicate their findings to a variety of stakeholders, including technical and non-technical audiences. Practice projects provide data scientists with the opportunity to develop these skills by requiring them to present their findings to stakeholders in a clear and concise manner.
For example, a data scientist working on a practice project to predict customer churn may need to present their findings to marketing and sales teams. They would need to be able to explain the model they developed, the data they used, and the insights they gained. They would also need to be able to answer questions and provide recommendations based on their findings.
Practice projects help data scientists develop the communication skills they need to be successful in their roles. By presenting their findings to stakeholders, data scientists can gain experience in communicating complex technical information in a clear and concise manner. This skill is essential for data scientists who want to be able to effectively collaborate with others and make an impact with their work.
Collaboration skills
Collaboration skills are essential for data scientists as they often work on projects that require input from a variety of stakeholders, including other data scientists, engineers, product managers, and business leaders. Practice projects provide data scientists with the opportunity to develop these skills by requiring them to collaborate with others on all aspects of the project, from data collection and analysis to model building and deployment.
For example, a data scientist working on a practice project to develop a predictive model for customer churn may need to collaborate with a product manager to understand the business goals of the project, with an engineer to build the data pipeline, and with a marketing team to interpret the results of the model. By working with others, data scientists can gain experience in communicating their ideas clearly, resolving conflicts, and working towards a common goal.
Collaboration skills are also important for data scientists who want to be successful in their careers. Data science is a team sport, and data scientists who are able to collaborate effectively with others are more likely to be successful in their projects and advance their careers.
Portfolio building
In the context of data science, a portfolio is a collection of work that demonstrates a data scientist’s skills and experience. Practice projects are an excellent way for data scientists to build their portfolio by providing them with hands-on experience in all aspects of the data science process, from data collection and cleaning to analysis and interpretation.
- Diverse projects: Practice projects allow data scientists to work on a variety of projects, showcasing their ability to solve different types of problems and apply their skills to different domains.
- End-to-end experience: Practice projects provide data scientists with the opportunity to work on projects from start to finish, giving them experience in all stages of the data science process.
- Real-world relevance: Practice projects often involve working on real-world problems, giving data scientists the opportunity to apply their skills to practical problems and gain valuable experience.
- Presentation and communication: Practice projects often require data scientists to present their findings to stakeholders, giving them the opportunity to develop their communication and presentation skills.
Overall, practice projects are a valuable way for data scientists to build their portfolio and showcase their skills. By working on practice projects, data scientists can gain experience in all aspects of the data science process, work on a variety of projects, and develop their communication and presentation skills.
FAQs on Data Science Practice Projects
Data science practice projects are an essential part of a data scientist’s learning and development journey. They provide valuable hands-on experience, help develop problem-solving skills, and allow data scientists to build their portfolio. However, there are also some common questions and misconceptions surrounding data science practice projects.
Question 1: What are the benefits of working on data science practice projects?
Answer: Data science practice projects offer numerous benefits, including hands-on experience, development of problem-solving skills, domain knowledge, communication skills, collaboration skills, and portfolio building.
Question 2: How do I choose a data science practice project?
Answer: When choosing a data science practice project, consider your interests, skills, and career goals. It’s also helpful to look for projects that are aligned with current industry trends and that provide opportunities to learn new technologies or techniques.
Question 3: How long should I spend on a data science practice project?
Answer: The amount of time spent on a data science practice project can vary depending on the complexity of the project. However, it’s important to set realistic expectations and allocate sufficient time to complete the project thoroughly.
Question 4: How can I get feedback on my data science practice projects?
Answer: There are several ways to get feedback on your data science practice projects. You can share your work with mentors, colleagues, or online communities. You can also participate in hackathons or competitions to get feedback from experts.
Question 5: How can I use data science practice projects to advance my career?
Answer: Data science practice projects can be used to advance your career in several ways. They can help you develop new skills, build your portfolio, and demonstrate your abilities to potential employers or clients.
Question 6: What are some common challenges associated with data science practice projects?
Answer: Some common challenges associated with data science practice projects include data availability, data quality, and computational resources. It’s important to carefully consider these challenges and plan accordingly.
Summary of key takeaways or final thought: Data science practice projects are an essential part of a data scientist’s learning and development journey. They provide valuable hands-on experience, help develop problem-solving skills, and allow data scientists to build their portfolio. By carefully choosing and executing data science practice projects, individuals can advance their careers and succeed in the field.
Transition to the next article section: For more information on data science practice projects, please refer to the following resources:
Tips for Data Science Practice Projects
Data science practice projects are an essential part of a data scientist’s learning and development journey. They provide valuable hands-on experience, help develop problem-solving skills, and allow data scientists to build their portfolio. Here are a few tips to help you get the most out of your data science practice projects:
Tip 1: Choose a project that is aligned with your interests and career goals. This will help you stay motivated and engaged throughout the project. If you are interested in a particular industry or domain, look for projects that are related to that area.
Tip 2: Start with a small project. This will help you get your feet wet and learn the basics of data science. As you gain more experience, you can take on more complex projects.
Tip 3: Set realistic expectations. Don’t try to do too much in one project. It’s better to complete a smaller project well than to start a larger project and not finish it.
Tip 4: Get feedback from others. Share your work with mentors, colleagues, or online communities. Getting feedback from others can help you improve your work and identify areas for improvement.
Tip 5: Document your work. This will help you keep track of your progress and make it easier to share your work with others.
Tip 6: Use the right tools and resources. There are a number of tools and resources available to help you with data science practice projects. Take advantage of these resources to make your work easier and more efficient.
Tip 7: Be patient and persistent. Data science practice projects can be challenging, but they are also very rewarding. Don’t give up if you don’t get the results you want right away. Keep working at it and you will eventually succeed.
Summary of key takeaways or benefits: By following these tips, you can increase your chances of success with data science practice projects. These projects are an essential part of a data scientist’s learning and development journey. They provide valuable hands-on experience, help develop problem-solving skills, and allow data scientists to build their portfolio.
Transition to the article’s conclusion: For more information on data science practice projects, please refer to the following resources:
Conclusion
Data science practice projects play a vital role in the learning and development of data scientists. They provide hands-on experience in all aspects of the data science process, from data collection and cleaning to analysis and interpretation. Through practice projects, data scientists develop essential skills such as problem-solving, communication, collaboration, and portfolio building.
In today’s data-driven world, data science practice projects are more important than ever before. Organizations are increasingly relying on data to make informed decisions, and data scientists are in high demand. By completing practice projects, data scientists can gain the skills and experience needed to succeed in this competitive field.
Youtube Video:
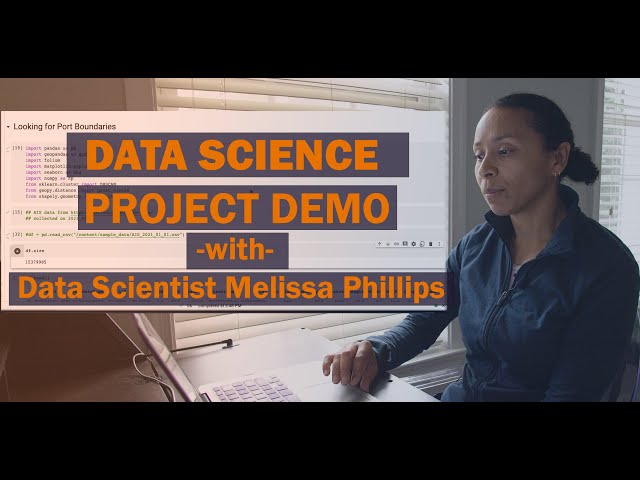