Project ideas for data science encompass a broad range of concepts, tools, and techniques employed to extract knowledge and insights from data. These projects delve into various domains, including data analysis, machine learning, and artificial intelligence, providing practical applications in fields such as healthcare, finance, and manufacturing.
The significance of project ideas for data science lies in their ability to bridge the gap between theoretical knowledge and practical implementation. By working on real-world projects, aspiring data scientists gain hands-on experience in data exploration, model building, and evaluation, refining their skills and enhancing their understanding of the field.
This article presents a comprehensive exploration of project ideas for data science, categorizing them based on difficulty level, domain, and specific techniques. We will delve into beginner-friendly projects suitable for those new to the field, intermediate-level projects that introduce more advanced concepts, and advanced projects that challenge experienced data scientists. Additionally, we will explore projects across various domains, showcasing the diverse applications of data science in different industries.
project ideas for data science
Project ideas for data science form the cornerstone of practical learning in the field. These ideas encompass various dimensions, each contributing to the development of essential skills and knowledge. Here are seven key aspects to consider when exploring project ideas for data science:
- Data Exploration: Uncover patterns and insights hidden within raw data.
- Data Visualization: Present data in a visually compelling manner to communicate insights effectively.
- Machine Learning Algorithms: Apply supervised and unsupervised learning techniques to uncover hidden patterns and make predictions.
- Model Evaluation: Assess the performance of machine learning models using appropriate metrics.
- Real-World Applications: Implement data science solutions to solve business problems and add value.
- Communication: Effectively communicate findings and insights to stakeholders.
- Ethical Considerations: Ensure responsible use of data and uphold ethical standards in data science practices.
These aspects are interconnected and essential for successful data science projects. For instance, effective data exploration helps identify the right machine learning algorithms to apply, while robust model evaluation ensures that the developed models perform as expected. Furthermore, considering ethical implications from the outset fosters trust and ensures that data science projects align with societal values.
Data Exploration
Data exploration lies at the heart of project ideas for data science, as it sets the foundation for uncovering valuable insights and patterns hidden within raw data. This process involves examining, cleaning, and transforming data to make it suitable for further analysis and modeling. Effective data exploration enables data scientists to gain a deep understanding of the data, identify potential relationships and trends, and formulate hypotheses for further investigation.
Consider a project idea for data science that aims to predict customer churn. Data exploration is crucial in this scenario, as it allows data scientists to understand the characteristics of customers who have churned in the past. By analyzing historical data, they can identify patterns and trends that may indicate factors contributing to churn, such as demographics, usage patterns, and customer satisfaction levels. This understanding helps in selecting appropriate machine learning algorithms for building predictive models and developing targeted interventions to reduce churn.
In summary, data exploration is an essential component of project ideas for data science, as it provides the foundation for understanding the data, uncovering patterns, and formulating hypotheses. By thoroughly exploring the data, data scientists can make informed decisions about the modeling approach and ultimately develop effective solutions to business problems.
Data Visualization
Data visualization plays a crucial role in project ideas for data science, as it enables effective communication of complex data and insights to stakeholders. By presenting data in visual formats, such as charts, graphs, and dashboards, data scientists can make their findings more accessible, understandable, and actionable.
- Clarity and Simplicity: Effective data visualizations prioritize clarity and simplicity, ensuring that the message is conveyed concisely and without overwhelming the audience with unnecessary details.
- Choosing the Right Chart Type: Selecting the appropriate chart type for the data is essential. Different chart types, such as bar charts, line charts, and scatterplots, are suited for different types of data and can highlight specific patterns and trends.
- Interactive Visualizations: Interactive visualizations allow users to explore the data and insights at their own pace. They can filter, sort, and drill down into the data, gaining a deeper understanding of the underlying patterns.
- Storytelling with Data: Data visualizations can be used to tell a compelling story, guiding the audience through the key findings and insights. By incorporating context and narrative, data scientists can make their presentations more engaging and persuasive.
In summary, data visualization is an integral part of project ideas for data science, enabling effective communication of complex data and insights. By carefully choosing the right chart types, designing clear and simple visuals, and incorporating interactivity, data scientists can ensure that their findings are understood and acted upon.
Machine Learning Algorithms
Machine learning algorithms are a fundamental component of project ideas for data science, as they enable data scientists to uncover hidden patterns, make predictions, and build intelligent systems. Supervised learning algorithms, such as linear regression and decision trees, learn from labeled data to make predictions, while unsupervised learning algorithms, such as clustering and dimensionality reduction, discover patterns in unlabeled data.
Consider a project idea for data science that aims to predict customer churn. Machine learning algorithms play a critical role in this project, as they can identify patterns and trends in customer behavior that may indicate a high risk of churn. By applying supervised learning algorithms to historical data, data scientists can train models that predict the likelihood of a customer churning. These models can then be used to develop targeted interventions to reduce churn, such as personalized offers or improved customer support.
In summary, machine learning algorithms are essential for project ideas for data science, as they provide the ability to uncover hidden patterns, make predictions, and build intelligent systems. By leveraging the power of machine learning, data scientists can develop innovative solutions to real-world problems and add value to businesses and organizations.
Model Evaluation
Model evaluation is an essential component of project ideas for data science, as it provides a quantitative assessment of the performance of machine learning models. By evaluating models using appropriate metrics, data scientists can determine how well the models perform on unseen data and identify areas for improvement.
Consider a project idea for data science that aims to predict customer churn. Model evaluation is crucial in this project, as it allows data scientists to assess the accuracy of the predictive model. By using metrics such as precision, recall, and F1-score, data scientists can determine how well the model predicts churned customers while minimizing false positives and false negatives. This evaluation helps in refining the model and ensuring that it meets the business requirements.
In summary, model evaluation is an integral part of project ideas for data science, as it enables data scientists to assess the performance of machine learning models and make informed decisions about model selection and deployment. By carefully selecting and interpreting evaluation metrics, data scientists can ensure that their models are reliable and effective in solving real-world problems.
Real-World Applications
In the realm of project ideas for data science, real-world applications hold immense significance. They provide a tangible connection between theoretical knowledge and practical problem-solving, enabling data scientists to leverage their skills to address real-world challenges and add value to businesses and organizations.
Consider a project idea that aims to optimize marketing campaigns for an e-commerce company. By analyzing customer data, purchase history, and campaign performance, data scientists can uncover patterns and insights that inform targeted marketing strategies. This data-driven approach helps the company identify high-value customers, personalize product recommendations, and allocate marketing budgets more effectively, leading to increased sales and improved customer satisfaction.
Real-world applications not only demonstrate the practical utility of data science but also foster collaboration between data scientists and business stakeholders. By working closely with domain experts, data scientists gain a deep understanding of the business context and can tailor their solutions to meet specific needs and objectives. This collaborative approach ensures that data science projects deliver tangible benefits and drive business outcomes.
Communication
In the context of project ideas for data science, effective communication plays a crucial role in bridging the gap between technical insights and actionable outcomes. Data scientists must be able to convey their findings and insights clearly and persuasively to stakeholders, including business leaders, project managers, and end-users.
Consider a project that involves developing a predictive model for customer churn. The data scientist responsible for this project must be able to explain the model’s underlying assumptions, limitations, and potential impact to stakeholders. This includes presenting the results in a non-technical manner, highlighting key insights and recommendations. Effective communication ensures that stakeholders understand the value of the data science project and can make informed decisions based on the findings.
Furthermore, effective communication fosters collaboration and buy-in from stakeholders. When stakeholders have a clear understanding of the project’s goals, methods, and outcomes, they are more likely to support and champion the initiative. This can lead to smoother project execution, increased adoption of data science solutions, and ultimately, greater business value.
Ethical Considerations
In the realm of project ideas for data science, ethical considerations play a pivotal role in guiding the responsible use of data and upholding ethical standards throughout the data science lifecycle. By integrating ethical considerations into project design and execution, data scientists can ensure that their work aligns with societal values, respects individual rights, and promotes fairness and transparency.
One key aspect of ethical considerations in data science projects is data privacy and confidentiality. Data scientists must adhere to privacy regulations and best practices to protect sensitive personal information. This includes obtaining informed consent from individuals before collecting and using their data, anonymizing data when appropriate, and implementing robust security measures to prevent unauthorized access.
Another important ethical consideration is algorithmic bias. Machine learning algorithms can perpetuate biases that exist in the data they are trained on, leading to discriminatory or unfair outcomes. Data scientists must carefully evaluate their algorithms for bias and take steps to mitigate it, such as using unbiased data sets, employing fairness metrics, and implementing algorithmic transparency techniques.
By incorporating ethical considerations into project ideas for data science, data scientists can contribute to building trust in data science and its applications. Ethical data science practices foster transparency, accountability, and responsible innovation, ensuring that data science projects deliver positive societal outcomes.
Frequently Asked Questions about Project Ideas for Data Science
This section addresses common questions and concerns regarding project ideas for data science, providing concise and informative answers to guide aspiring data scientists.
Question 1: What are some beginner-friendly project ideas for data science?
For those new to data science, beginner-friendly project ideas include data exploration and visualization projects using publicly available datasets. These projects can involve analyzing data to uncover patterns, trends, and insights, as well as creating visual representations of the data to communicate findings effectively.
Question 2: How do I choose a project idea that aligns with my interests and career goals?
Consider your interests and career aspirations when selecting a project idea. Explore different domains where data science is applied, such as healthcare, finance, or retail. Choose a project that aligns with your interests and provides opportunities to develop skills relevant to your career goals.
Question 3: Where can I find inspiration and resources for project ideas?
Numerous online resources and communities provide inspiration and resources for project ideas. Explore platforms like Kaggle, DrivenData, and GitHub for project ideas, datasets, and code snippets. Additionally, attend industry events, webinars, and conferences to network with data science professionals and gain insights into real-world applications.
Tips for Project Ideas in Data Science
Selecting and developing impactful project ideas is essential for aspiring data scientists. Here are several tips to guide you in this process:
Tip 1: Explore Real-World Problems
Identify real-world challenges that can be addressed using data science techniques. This approach ensures that your project has practical relevance and potential for societal impact.
Tip 2: Leverage Open-Source Resources
Take advantage of open-source datasets, libraries, and tools to accelerate your project development. These resources provide a wealth of materials to support your data exploration, modeling, and visualization tasks.
Tip 3: Collaborate with Domain Experts
Seek collaborations with experts in the domain you are targeting. Their insights can provide valuable context, help you refine your project goals, and ensure the relevance of your findings.
Tip 4: Prioritize Data Quality
Emphasize data quality throughout your project. Utilize data cleaning and preprocessing techniques to ensure the accuracy and reliability of your results. High-quality data leads to more robust and trustworthy models.
Tip 5: Choose Appropriate Metrics
Carefully select evaluation metrics that align with your project objectives. These metrics will serve as benchmarks to assess the performance and effectiveness of your data science models.
By following these tips, you can enhance the quality and impact of your project ideas in data science.
In conclusion, selecting a compelling project idea is crucial for a successful data science project. Consider the tips outlined above to identify a project that aligns with your interests, leverages available resources, and has the potential to drive meaningful outcomes.
Conclusion
Project ideas for data science encompass a wide range of concepts, tools, and techniques that empower data scientists to extract knowledge and insights from data. These projects provide practical experience in data exploration, model building, and evaluation, refining skills and enhancing understanding of the field. By exploring beginner-friendly, intermediate-level, and advanced projects across various domains, aspiring data scientists can develop their expertise and contribute to the advancement of data science.
The key to successful project ideas lies in identifying problems that can be addressed using data science techniques, leveraging open-source resources, collaborating with domain experts, prioritizing data quality, and choosing appropriate metrics. By following these principles, data scientists can develop impactful projects that drive meaningful outcomes and contribute to the advancement of the field.
Youtube Video:
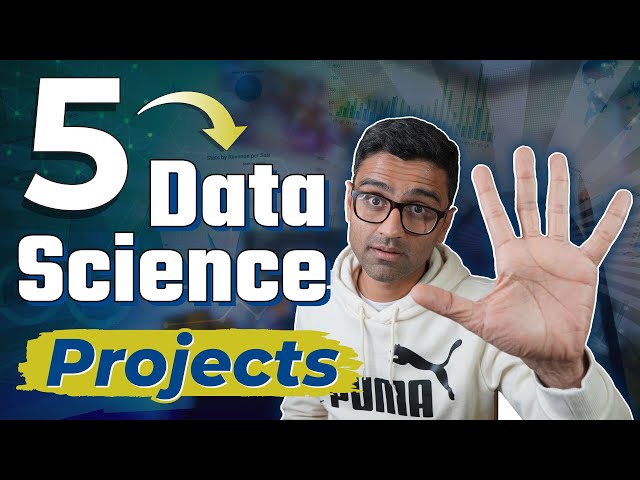